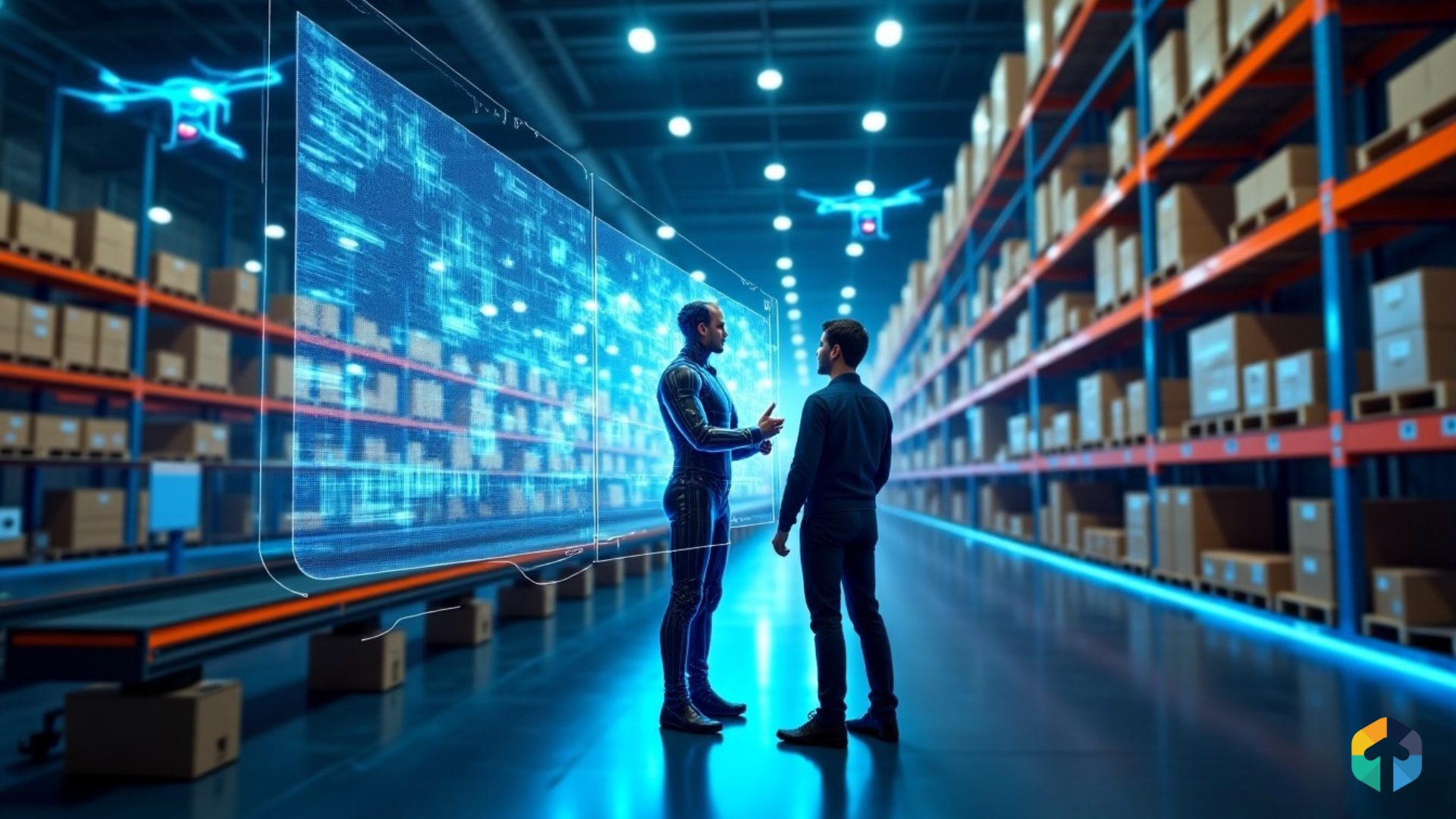
The Inventory Balancing Challenge
Inventory management remains one of the most persistent challenges in retail and manufacturing operations. Too much inventory ties up capital and warehouse space while risking obsolescence too little leads to stockouts, disappointed customers, and lost sales.
Table of Contents
This delicate balancing act becomes increasingly complex as businesses face fluctuating demand patterns, supply chain disruptions, and heightened customer expectations for immediate availability.
Traditional inventory approaches often rely on simple par levels or basic reorder points that fail to capture market dynamics or seasonal variations.
Inventory managers frequently find themselves reacting to problems, rushing emergency orders for depleted items, and marking down overstocked products rather than proactively optimizing stock levels.
Meanwhile, stock shrinkage from theft, damage, and administrative errors continues to erode already-thin margins.
Industry research suggests that retailers typically experience out-of-stock rates between 8% and 12% while simultaneously carrying weeks of unnecessary inventory in other categories.
This inefficiency creates significant costs, including excess carrying expenses, emergency shipping charges, lost sales, and diminished customer loyalty.
Understanding AI Agent Inventory Intelligence
AI inventory management systems represent a fundamental shift from reactive to predictive approaches. These systems leverage advanced algorithms, machine learning, and multiple data inputs to create dynamic, self-improving inventory optimization across complex product assortments.
Unlike basic inventory software that simply tracks current quantities, AI-powered systems analyze complex patterns in historical data, identify correlations with external factors like weather or events, and continuously improve their forecasting accuracy through feedback loops.
The technology functions as both an early warning system for potential problems and an optimization engine for capital efficiency.
Organizations implementing these systems report substantial improvements in inventory performance metrics. Retailers experience fewer stock-outs while simultaneously reducing overall inventory investment.
Manufacturers maintain production continuity with lower raw material holdings. Distribution centers operate more efficiently with optimized stock positions.
Smarter Inventory Starts Here
See how AI helps you reduce stock-outs, cut cost, and keep your best sellers in stock without the guess work

Key Capabilities Enhancing Inventory Performance
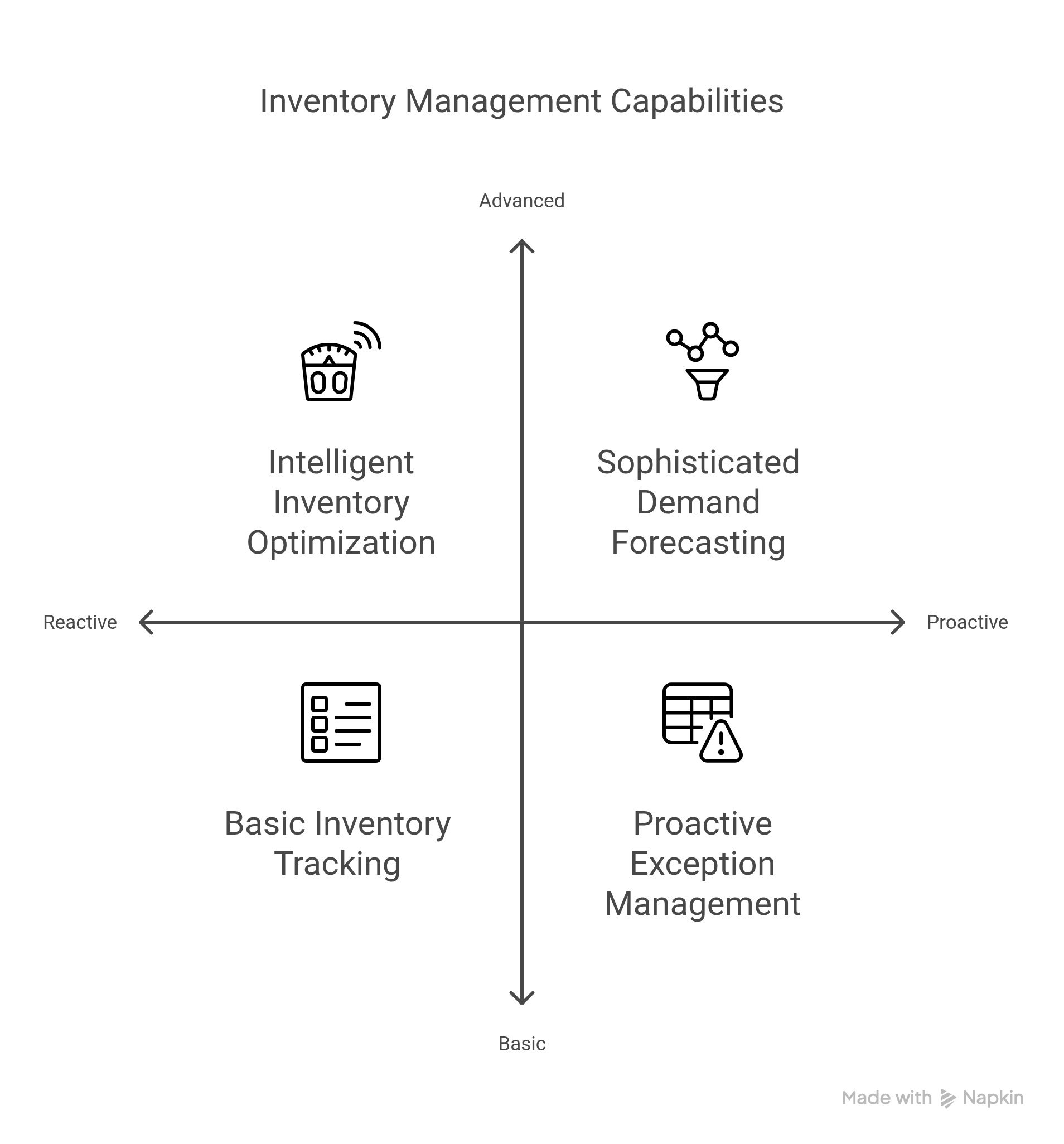
Sophisticated Demand Forecasting
The core intelligence lies in the system's ability to predict future inventory needs with remarkable accuracy.
Analyzes historical sales patterns at granular levels.
Identifies seasonal trends and cyclical demand patterns.
Incorporates external factors like weather, events, and economic indicators.
Recognizes correlations between seemingly unrelated products.
Adapts quickly to emerging trends and pattern shifts.
Differentiates between regular demand and anomalies.
This forecasting capability operates at multiple levels, from individual SKUs at specific locations to broad category trends across regions, creating nuanced predictions that account for local variations while leveraging aggregate insights.
Retailers with diverse store footprints particularly value how the system recognizes location-specific patterns, understanding that the same product might sell differently in urban stores versus suburban locations, or in regions with different weather patterns and cultural preferences.
Intelligent Inventory Optimization
Beyond simply predicting demand, the system optimizes inventory positioning:
Recommends optimal stock levels by location and time period.
Balances inventory investment against service level targets.
Calculates safety stock requirements based on supply variability.
Identifies slow-moving inventory requiring attention.
Suggests cross-location transfers to avoid stockouts.
Recommends assortment changes based on performance.
This optimization capability transforms inventory from a reactive function to a strategic advantage. By right-sizing inventory across thousands of SKUs, businesses maintain availability with substantially less capital investment.
Distribution operations benefit from the system's ability to differentiate between items requiring different inventory approaches. Fast-moving, predictable products might operate with minimal safety stock, while critical items with irregular demand patterns maintain higher buffers to ensure availability.
Proactive Exception Management
The system actively monitors for inventory anomalies requiring attention:
Alerts when items are trending toward stockout.
Identifies unusual movement patterns potentially indicating theft.
Flag discrepancies between the recorded and actual inventory.
Notices sudden demand changes requiring intervention.
Highlights potential quality issues through return pattern analysis.
Detects administrative errors in receiving or transferring.
This exception management capability transforms loss prevention from periodic inventory counts to continuous monitoring.
Rather than discovering shrinkage weeks or months after it occurs, the system identifies suspicious patterns immediately, allowing for timely investigation.
Retail operations find this particularly valuable for high-value items with significant shrink risk.
The system might notice that a location is selling premium items at unusually high rates without corresponding decreases in recorded inventory, suggesting potential theft requiring investigation.
Measurable Business Impact Across Operations
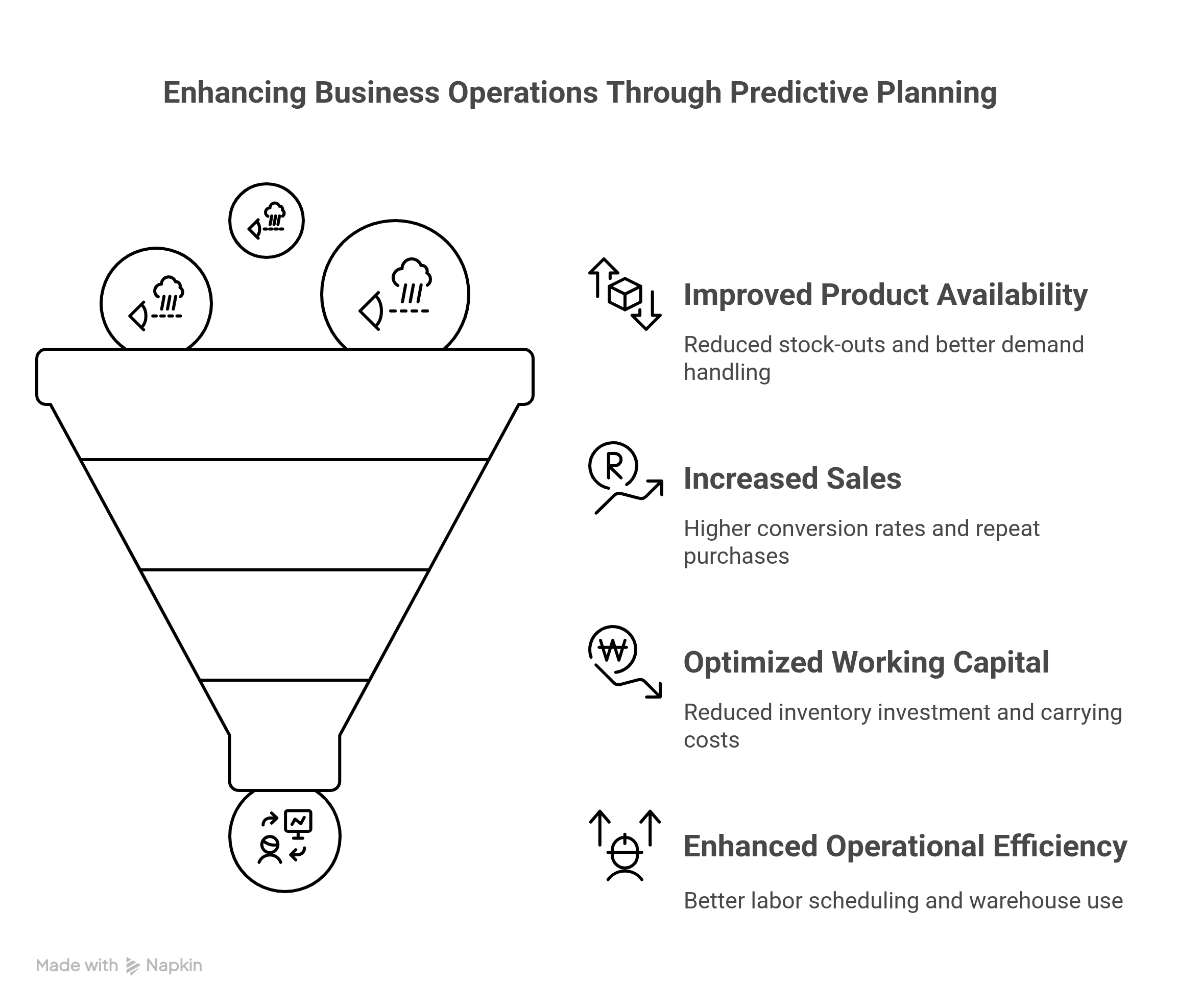
Improved Product Availability and Sales
The most direct benefit comes through improved in-stock positions:
Reduced stock-out frequency across product assortments.
Fewer lost sales due to unavailable merchandise.
Better handling of demand spikes and seasonal transitions.
More responsive adaptation to changing consumer preferences.
Improved customer satisfaction and repeat purchase behavior.
Higher conversion rates from browsers to buyers.
Retail businesses typically experience sales increases simply through better availability of high-demand items.
When customers consistently find what they're looking for, both immediate sales and long-term loyalty improve.
Consumer electronics retailers report particular benefits during product transitions and high-demand periods.
The system's ability to predict which new products will gain traction allows for more responsive inventory positioning, capturing sales that would otherwise be lost to competitors.
Significant Working Capital Optimization
Beyond sales improvements, businesses experience substantial financial benefits.
Reduced overall inventory investment.
Lower carrying costs for warehouse space and handling.
Decreased waste from obsolete or expired products.
Minimized emergency shipping and expediting expenses.
Improved cash flow through inventory efficiency.
Better allocation of limited open-to-buy dollars.
Manufacturing operations report some of the strongest financial impacts, as raw material and work-in-progress inventories often represent significant capital investments.
By maintaining production continuity with lower average inventory levels, manufacturers improve both asset utilization and cash position.
Seasonal businesses particularly value how the system helps manage transitions between periods.
Rather than the common problem of ending seasons with excess inventory requiring markdown, the system tapers ordering appropriately while maintaining the availability of core products.
Operation Efficiency Through Better Planning
The predictive capabilities create substantial operational improvements
More efficient labor scheduling based on anticipated activity.
Better coordination between purchasing and merchandising.
Reduced time spent on manual inventory adjustments.
More productive use of warehouse space.
Smoother receiving and fulfillment operations.
Decreased time spent resolving inventory discrepancies.
Distribution centers experience significant efficiency gains through more predictable workflows. When inventory arrives and departs according to accurate forecasts, labor and equipment utilization improve while overtime decreases.
The Ultimate Guide to Hiring FlutterFlow Developers
Looking to build a powerful app with FlutterFlow but need the right talent?
This guide walks you through everything you need to know to hire skilled
FlutterFlow developers for your project.
Read more
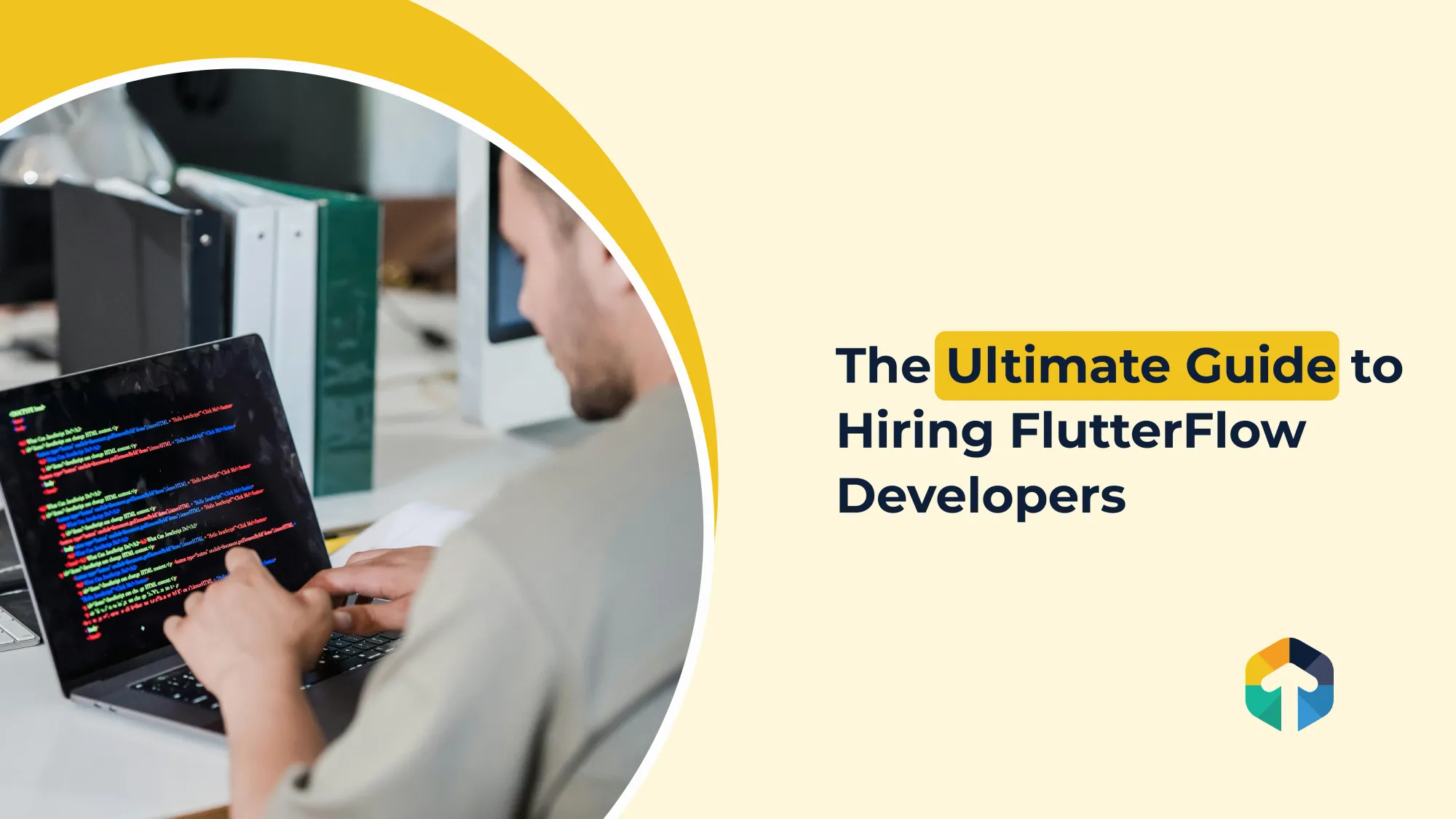
Implementation Considerations for Organizations
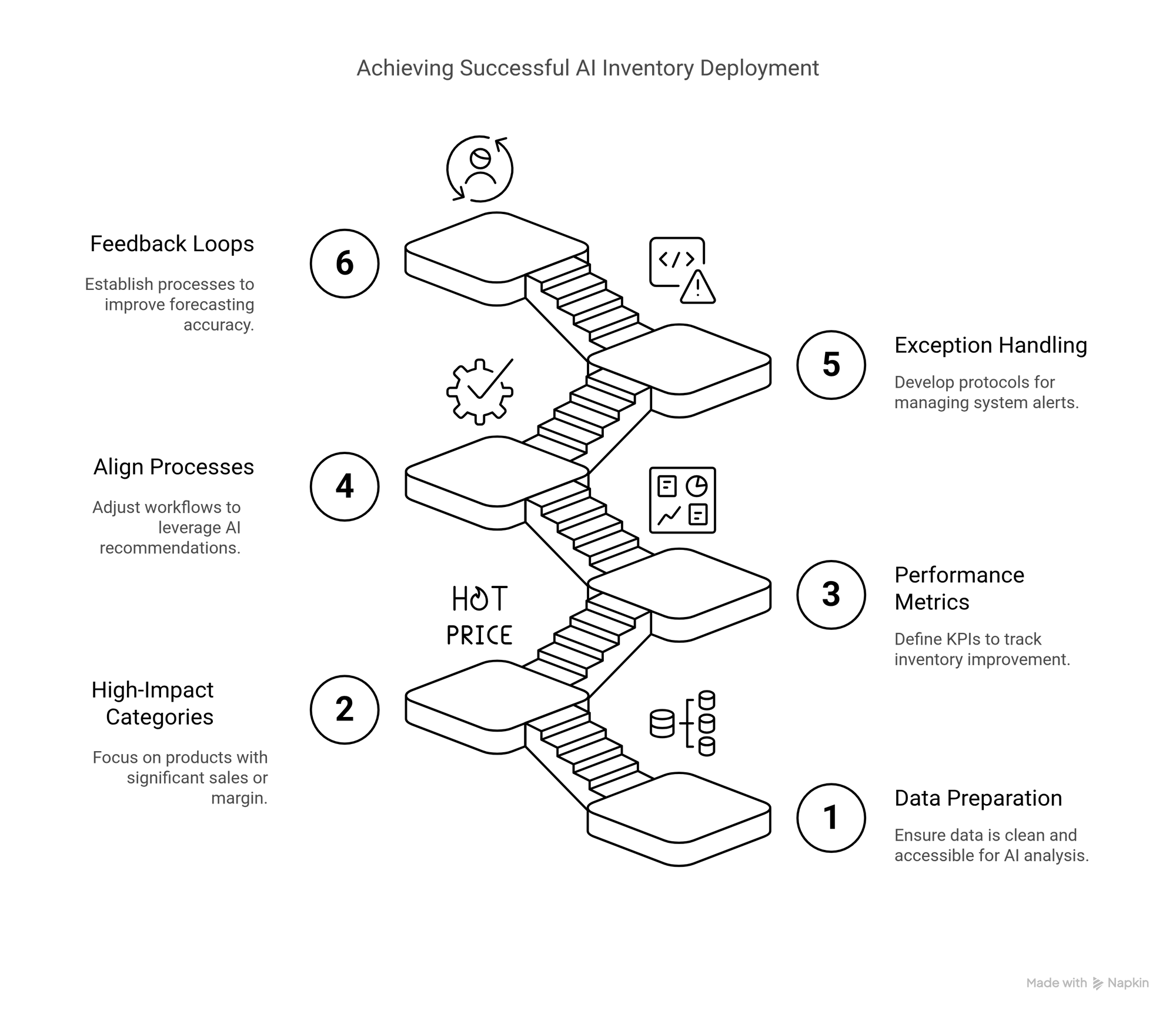
While benefits are compelling, successful deployment requires thoughtful planning.
Begin with data preparation - Ensure historical inventory and sales data are clean and accessible.
Start with high-impact categories - Focus initially on products with significant sales volume or margin contribution.
Establish clear performance metrics - Define specific inventory KPIs to track improvement.
Align organizational processes - Adjust purchasing and planning workflows to leverage system recommendations.
Plan for exception handling - Develop clear protocols for managing system alerts and anomalies.
Create feedback loops - Establish processes to capture outcomes and continuously improve forecasting accuracy.
Organizations that follow these implementation practices typically see faster adoption and stronger results from their AI inventory investments.
Smarter Inventory Starts Here
See how AI helps you reduce stock-outs, cut cost, and keep your best sellers in stock without the guess work

Future Developments in Inventory Intelligence
As these technologies continue to evolve, organizations can anticipate several promising enhancements.
Computer vision integration will enable real-time shelf monitoring in retail environments.
Supply chain visibility will incorporate vendor production and shipping data into forecasts.
Dynamic pricing integration will optimize both inventory and margin simultaneously.
Sustainability optimization will balance inventory efficiency with environmental impact.
Extended supplier collaboration will automate replenishment across the supply chain.
Forward-thinking organizations are already preparing for these capabilities by building strong foundations with current-generation systems.
Conclusion
AI-powered inventory management transforms what has traditionally been viewed as a necessary operational function into a source of competitive advantage.
By applying advanced analytics and machine learning to the fundamental challenge of matching supply with demand, these systems enable businesses to satisfy customers more completely while employing less capital.
For retail, manufacturing, and distribution organizations seeking to thrive in an increasingly volatile market environment, intelligent inventory management offers a proven path to improved performance.
As consumer expectations continue to rise and supply chains face ongoing challenges, the ability to optimize inventory positions will increasingly separate market leaders from lagging competitors.
If you are looking integrate AI in your inventory management system we can help you with seamless integration and help you grow your business with improved efficiency. Contact us today!
FAQs
How quickly can organizations expect to see results?
Most businesses see measurable improvements in key metrics within the first inventory turn after implementation, with benefits accelerating as the system accumulates data and refines its algorithms.
How does the system handle new products without sales history?
Advanced systems can forecast new product demand based on attributes of similar items, launch parameters, and initial sales patterns, becoming increasingly accurate as data accumulates.
Can the system adapt to major disruptions like those seen during recent supply chain crises?
While no system can perfectly predict unprecedented disruptions, AI inventory systems typically adapt faster than traditional approaches, quickly incorporating new patterns and identifying alternative supply options.
How much historical data is needed for accurate forecasting?
Most systems perform reasonably well with one year of data and improve substantially with two to three years of history, though even limited data provides better results than non-AI approaches..
How does the system handle products with highly variable demand?
Rather than treating all products the same, advanced systems apply different forecasting models to different demand patterns, using appropriate statistical approaches for stable versus highly variable items.